Files
Download Full Text (746 KB)
Description
Alzheimer’s disease (AD) is the most common form of dementia and one of the most prominent challenges of precision healthcare is early identification of AD. To combat this latency in diagnosis, integration of machine learning has been exercised for more cost efficient and powerful diagnostic tools. Specifically, we have developed a workflow for identifying AD within a given sample. Utilizing cerebral cortex proteomic data as a baseline, we were able to test two different forms of feature selection and 6 different machine learning methods. The best performing of these combinations was using a Support Vector Machine (SVM) method with features selected from Maximum Relevance Minimum Redundancy (MRMR) . This method had an average accuracy of 93.25% across and had yielded relatively good precision across 100 iterations. Furthering these types of predictions methods could allow a better quality and longevity of life for those at risk of Alzheimer's Disease.
Funding: Funding for this project was supplied by ND EPSCoR STEM (UND0025726), the American Society for Pharmacology & Experimental Therapeutics (ASPET) SURF Program, the Chair of the Department of Biomedical Sciences, the Division of Research & Economic Development at the University of North Dakota, an Institutional Development Award (IDeA) from the National Institute of General Medical Sciences of the National Institutes of Health under grant number P20GM103442, and the Dean of the University of North Dakota School of Medicine & Health Sciences.
Publication Date
12-15-2021
Document Type
Poster
Recommended Citation
Rowbotham, Kincaid; Li, Ling; and Wang, Xusheng, "Building the Path to Early Alzheimer's Prediction Using Machine Learning" (2021). Arts & Sciences Undergraduate Showcase. 5.
https://commons.und.edu/as-showcase/5
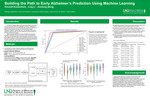
Comments
Presented at the Fall 2021 Virtual UNDergraduate Showcase, Grand Forks, ND, December 9, 2021.